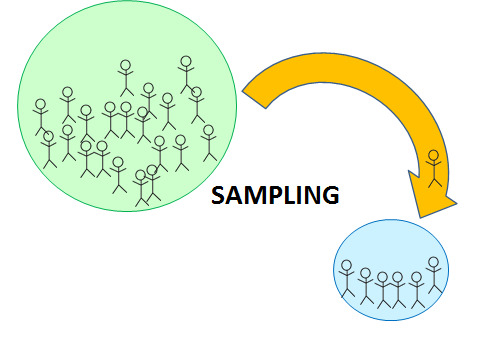
There is practical difficulty in studying all the individuals in the population and it is too time-consuming not cost effective.
Sample results are usually acceptable if the sample is representative of the population to be studied
The main Objectives of Sampling are:
Estimation of population parameters (Mean, proportion etc.) from the sample statistics.
To test the hypothesis about the population from which the sample or samples are drawn,
SAMPLING FRAME: Is a listing of the members of the universe form which the sample is to be drawn
The completeness of the sampling frame influences the quality of the sample drawn from it.
Sampling methods
Simple random sampling
Systematic random sampling
Stratified random sampling
Multistage sampling
Multi-phase sampling
Cluster sampling
Simple Random Sampling
This is done by
Assigning a number to each of the units in the sampling frame.
The numbers are randomly chosen to determine which units are to be included in to sample.
Each unit has an equal chance of being drawn in the sample.
For ensuring that the Numbers are Chosen Randomly, one can use
a random number table, Lottery method or the numbers on currency notes
Advantages
It provides us with a sample that is highly representative of the population being studied, as it avoids human bias
Hence it allows us to make generalizations from the sample to the population.
Disadvantages
A simple random sample can only be carried out if the list of the population is available and is complete.
Attaining a complete list of the population can be difficult
Due to privacy policies or require a lengthy process to attain permissions.
There may be no single list detailing the population you are interested in.
Even if the list has all the contact details of potential participants, contacting them may be challenging, as the sample may be geographically scattered.
Systematic random sampling
It consists of the following steps
(a) Defining the population (p);
(b) Deciding on the sample size (s);
(c) Listing the population and assigning numbers to cases (sampling frame);
(e) Calculating the ‘sampling interval’ (p/s = K); Total Population size/desired sample size = Sampling interval (K)
(f) Selecting the first unit RANDOMLY; and
(g) Selecting every Kth unit from there
The first unit is selected randomly and then every Kth unit is selected.
For example: For a study among the households of a locality.
- The houses are numbered first.
- Then for a 10% sample, a random number is selected between 1&10 (say four).
- Then every 10th number is selected form that point e.g. 4.14,24 34 etc.
The ‘regular interval (K)’ depends upon the size of the sample to be withdrawn and is known as the ‘Sampling interval’.
Advantages
Systemic random sample also provides us with a sample that is representative of the population under study
Relative to the simple random sample, it may be more convenient when the population is large, scattered and not homogeneous
Disadvantages
A systematic random sample can be carried out only if a complete list of the population is available
If the list of the population has some kind of periodic arrangement (pattern), systematic sampling could pick out similar cases rather than completely random ones
Stratified random sampling
The sample is drawn in a way so that each portion of the sample represents corresponding strata of the study population.
This method is useful when one is interested in analyzing the data by a certain characteristic of the population viz religion, socioeconomic strata.
The steps in stratified random sampling are:
To split the population into strata, i.e. sections or segments.
The strata are chosen to divide a population into important categories relevant to the research interest
The sample is then drawn from each stratum
Randomly
In proportion to its size
For example, if want to find out the prevalence of anemia in school students,
Split schools into rural, urban, and peri-urban as the prevalence may be quite distinct between these regions.
The second step is to take a simple random sample within each stratum in proportion to the numbers enrolled in the schools in each stratum
Multistage sampling
The sampling procedures carried out in several stages
Each stage uses random sampling techniques.
This is employed in large, countrywide surveys.
In the first stage, random numbers of districts are chosen in all the states,
followed by random no. of Talukas,
From among the chosen Taluks, villages are chosen randomly.
E.g. For hookworm survey in school children in a district.
Choose 10% of Talukas randomly and then
Choose10% of the villages situated in the chosen Talukas
Among the chosen villages, choose 10% of the schools randomly
From the chosen schools, 10% of the students are selected randomly, yielding a 10% sample of the district school children
Multi-phase sampling
In this method, part of the information is collected from the whole population& part, from the sub- sample.
E.g. In a TB survey,
Mantoux test may be done in all subjects of the study population in the first phase;
In the second phase, Mantoux positive cases undergo x-ray of the chest
Among the x-ray positive cases, sputum may be examined in the third phase.
Cluster sampling
This sampling technique used when a large study population is "naturally" divided into clusters and the clusters in turn have a homogeneous population
E.g. the country is sub-divided into towns, cities, wards, villages etc. but relatively homogeneous groupings are evident in these villages, slums etc.
In this technique, the total population of each cluster must be known
The complete list (sampling frame) of all the individuals in the country is not necessary
A fixed number of clusters are chosen using cluster sampling
A small sample is then selected from the chosen clusters using simple random sampling OR
The entire population of the cluster may be surveyed
The advantage of cluster sampling is that it is cheap, quick, and easy as Instead of sampling the entire country when using simple random sampling, the researcher can allocate resources to the few selected clusters
The disadvantage of Cluster Sampling is that it is the least representative of the population out of all the types of probability samples
E.g. WHO has approved ‘30 – cluster sampling’ for evaluating the immunization coverage among 12-23 month old children in the entire country
In this method only 210 children (7 from each of the 30 chosen clusters) are to be examined and the findings can be generalized to be representative of the country’s immunization coverage among 12 – 23 months old children
Sampling Errors
Sampling error: the difference between a sample statistic and its corresponding population parameter.
If a number of samples are taken from the same population, the statistics like mean and SD will be slightly different in each sample
This type of variation from sample to sample and sample to the population is called sampling error
Since only part (sample) of the population is examined instead of the whole, this error is inherent in the sampling process
The factors affecting the sampling error are
The size of the sample (as the size of the sample increases sampling error decreases)
The natural variability of the individual readings
Non – Sampling Errors
This error has no relationship to the sampling technique
The main reasons are:
- human-related data recording (observer variation)
- Inadequate calibration of the instruments
- non-response from the sample subjects
These errors are more important than the sampling errors and measures should be taken to minimize these